In this tutorial, you will learn how to mask images using OpenCV.
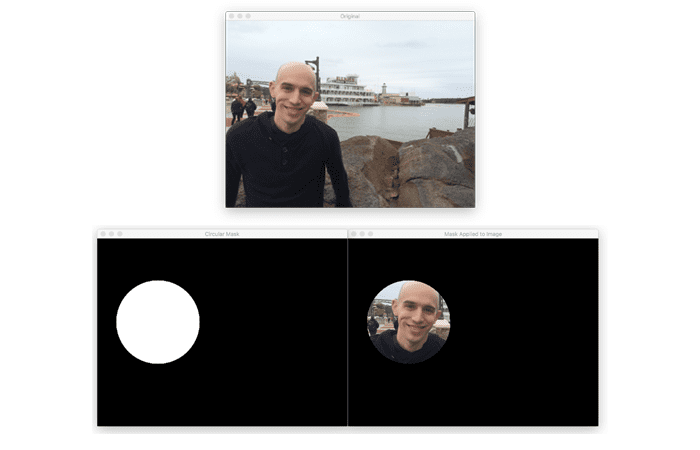
My previous guide discussed bitwise operations, a very common set of techniques used heavily in image processing.
And as I hinted previously, we can use both bitwise operations and masks to construct ROIs that are non-rectangular. This allows us to extract regions from images that are of completely arbitrary shape.
Put simply; a mask allows us to focus only on the portions of the image that interests us.
For example, let’s say that we were building a computer vision system to recognize faces. The only part of the image we are interested in finding and describing is the parts of the image that contain faces — we simply don’t care about the rest of the image’s content. Provided that we could find the faces in the image, we may construct a mask to show only the faces in the image.
Another image masking application you’ll encounter is alpha blending and transparency (e.g., in this guide on Creating GIFs with OpenCV). When applying transparency to images with OpenCV, we need to tell OpenCV what parts of the image transparency should be applied to versus not — masks allow us to make that distinction.
To learn how to perform image masking with OpenCV, just keep reading.
Image Masking with OpenCV
In the first part of this tutorial, we’ll configure our development environment and review our project structure.
We’ll then implement a Python script to mask images with OpenCV.
Configuring your development environment
To follow this guide, you need to have the OpenCV library installed on your system.
Luckily, OpenCV is pip-installable:
$ pip install opencv-contrib-python
If you need help configuring your development environment for OpenCV, I highly recommend that you read my pip install OpenCV guide — it will have you up and running in a matter of minutes.
Having problems configuring your development environment?
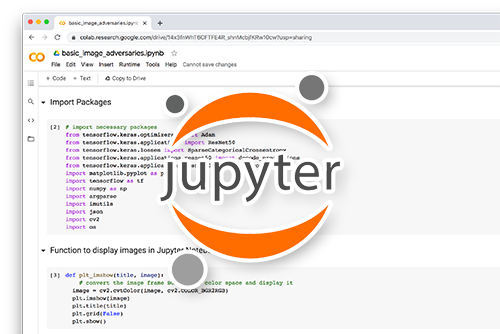
All that said, are you:
- Short on time?
- Learning on your employer’s administratively locked system?
- Wanting to skip the hassle of fighting with the command line, package managers, and virtual environments?
- Ready to run the code right now on your Windows, macOS, or Linux systems?
Then join PyImageSearch Plus today!
Gain access to Jupyter Notebooks for this tutorial and other PyImageSearch guides that are pre-configured to run on Google Colab’s ecosystem right in your web browser! No installation required.
And best of all, these Jupyter Notebooks will run on Windows, macOS, and Linux!
Project structure
Performing image masking with OpenCV is easier than you think. But before we write any code, let’s first review our project directory structure.
Start by using the “Downloads” section of this guide to access the source code and example image.
Your project folder should look like the following:
$ tree . --dirsfirst . ├── adrian.png └── opencv_masking.py 0 directories, 2 files
Our opencv_masking.py
script will load the input adrian.png
image from disk. We’ll then use masking to extract both the body and face from the image using rectangular and circular masks, respectively.
Implementing image masking with OpenCV
Let’s learn how to apply image masking using OpenCV!
Open the opencv_masking.py
file in your project directory structure, and let’s get to work:
# import the necessary packages import numpy as np import argparse import cv2 # construct the argument parser and parse the arguments ap = argparse.ArgumentParser() ap.add_argument("-i", "--image", type=str, default="adrian.png", help="path to the input image") args = vars(ap.parse_args())
Lines 2-4 import our required Python packages. We then parse our command line arguments on Lines 7-10.
We only need a single switch here, --image
, which is the path to the image we want to mask. We go ahead and default the --image
argument to the adrian.png
file in our project directory.
Let’s now load this image from disk and perform masking:
# load the original input image and display it to our screen image = cv2.imread(args["image"]) cv2.imshow("Original", image) # a mask is the same size as our image, but has only two pixel # values, 0 and 255 -- pixels with a value of 0 (background) are # ignored in the original image while mask pixels with a value of # 255 (foreground) are allowed to be kept mask = np.zeros(image.shape[:2], dtype="uint8") cv2.rectangle(mask, (0, 90), (290, 450), 255, -1) cv2.imshow("Rectangular Mask", mask) # apply our mask -- notice how only the person in the image is # cropped out masked = cv2.bitwise_and(image, image, mask=mask) cv2.imshow("Mask Applied to Image", masked) cv2.waitKey(0)
Lines 13 and 14 load the original image
from disk and display it to our screen:
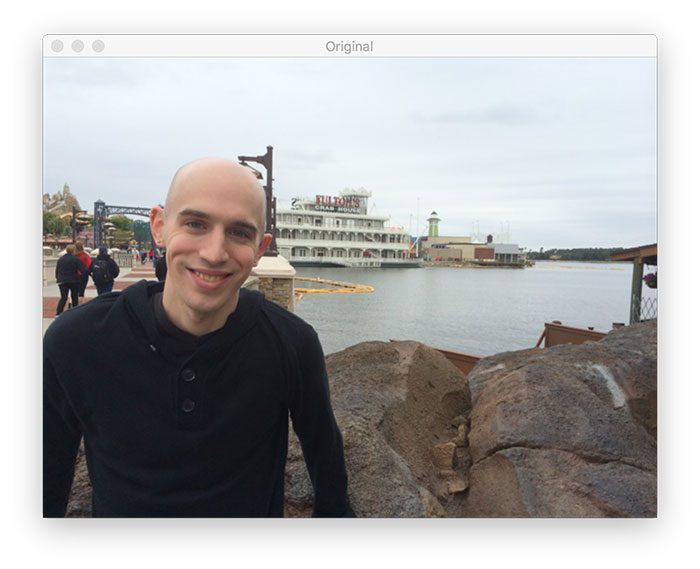
We then construct a NumPy array, filled with zeros, with the same width and height as our original image on Line 20.
As I mentioned in our previous tutorial on Image cropping with OpenCV, we can use object detection methods to detect objects/people in images automatically. Still, we’ll be using our a priori knowledge of our example image for the time being.
We know that the region we want to extract is in the image’s bottom-left corner. Line 21 draws a white rectangle on our mask, which corresponds to the region we want to extract from our original image.
Remember reviewing the cv2.bitwise_and
function in our bitwise operations tutorial? It turns out that this function is used extensively when applying masks to images.
We apply our mask on Line 26 using the cv2.bitwise_and
function.
The first two parameters are the image
itself (i.e., the image where we want to apply the bitwise operation).
However, the important part of this function is the mask
keyword. When supplied, the bitwise_and
function is True
when the pixel values of the input images are equal, and the mask is non-zero at each (x, y)-coordinate (in this case, only pixels that are part of the white rectangle).
After applying our mask, we display the output on Lines 27 and 28, which you can see in Figure 3:
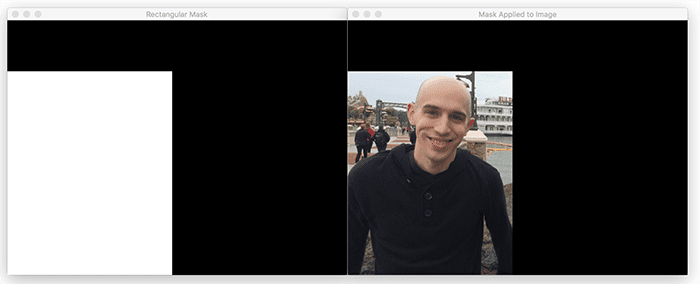
Using our rectangular mask, we could extract only the region of the image that contains the person and ignore the rest.
Let’s look at another example, but this time using a non-rectangular mask:
# now, let's make a circular mask with a radius of 100 pixels and # apply the mask again mask = np.zeros(image.shape[:2], dtype="uint8") cv2.circle(mask, (145, 200), 100, 255, -1) masked = cv2.bitwise_and(image, image, mask=mask) # show the output images cv2.imshow("Circular Mask", mask) cv2.imshow("Mask Applied to Image", masked) cv2.waitKey(0)
On Line 32, we re-initialize our mask
to be filled with zeros and the same dimensions as our original image.
Then, we draw a white circle on our mask image, starting at the center of my face with a radius of 100
pixels.
Applying the circular mask is then performed on Line 34, again using the cv2.bitwise_and
function.
The results of our circular mask can be seen in Figure 4:
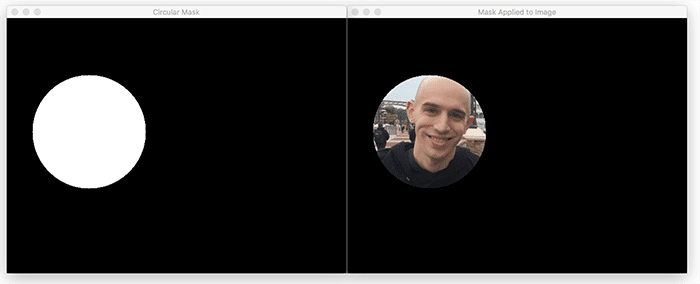
Here, we can see that our circle mask is shown on the left and the application of the mask on the right. Unlike the output from Figure 3, when we extracted a rectangular region, this time, we have extracted a circular region that corresponds to only my face in the image.
Furthermore, we can use this approach to extract regions from an image of arbitrary shape (rectangles, circles, lines, polygons, etc.).
OpenCV image masking results
To perform image masking with OpenCV, be sure to access the “Downloads” section of this tutorial to retrieve the source code and example image.
From there, open a shell and execute the following command:
$ python opencv_masking.py
Your masking output should match mine from the previous section.
What’s next?
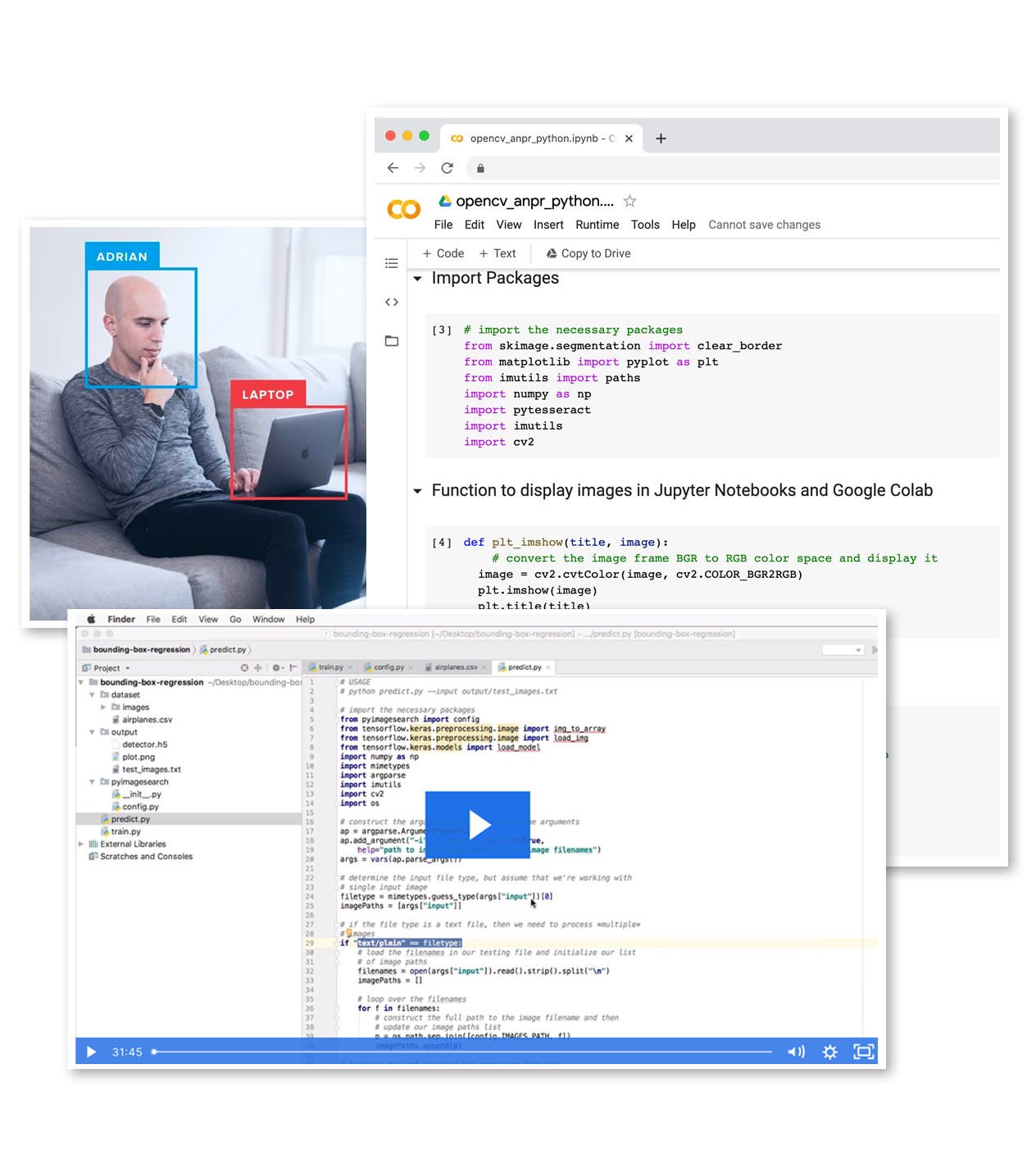
Would you enjoy learning how to successfully and confidently apply OpenCV to your projects?
Are you worried that configuring your development environment for Computer Vision, Deep Learning, and OpenCV will be too challenging, resulting in confusing, hard to debug error messages?
Concerned that you’ll get lost sifting through endless tutorials and video guides on your own as you struggle to master Computer Vision?
Great, because I’ve got you covered. PyImageSearch University is your chance to learn from me at your own pace.
You’ll find everything you need to master the basics (like we did together today) and move on to advanced concepts.
Don’t worry about your operating system or development environment. I’ve got you covered with pre-configured Jupyter Notebooks in Google Colab for every tutorial on PyImageSearch, including Jupyter Notebooks for our brand new weekly tutorials as well!
Best of all, these Jupyter Notebooks will run on your machine, regardless of whether you are using Windows, macOS, or Linux! Irrespective of the operating system used, you will still be able to follow along and run the code in every lesson (all inside the convenience of your web browser).
Additionally, you can massively accelerate your progress by watching our video lessons accompanying each post. Every lesson at PyImageSearch University includes a detailed, step-by-step video guide.
You may feel like learning Computer Vision, Deep Learning, and OpenCV is too hard. Don’t worry; I’ll guide you gradually through each lecture and topic, so we build a solid foundation, and you grasp all the content.
When you think about it, PyImageSearch University is almost an unfair advantage compared to self-guided learning. You’ll learn more efficiently and master Computer Vision faster.
Oh, and did I mention you’ll also get Certificates of Completion as you progress through each course at PyImageSearch University?
I’m sure PyImageSearch University will help you master OpenCV drawing and all the other computer vision skills you will need. Why not join today?
Summary
In this tutorial, you learned the basics of masking using OpenCV.
The key point of masks is that they allow us to focus our computation only on regions of the image that interest us. Focusing our computations on regions that interest us dramatically impacts when we explore topics such as machine learning, image classification, and object detection.
For example, let’s assume that we wanted to build a system to classify the species of the flower.
In reality, we are probably only interested in the flower petals’ color and texture to perform the classification. But since we are capturing the photo in a natural environment, we’ll also have many other regions in our image, including dirt from the ground, insects, and other flowers crowding the view. How will we quantify and classify just the flower we are interested in? As we’ll see, the answer is masks.
To download the source code to this post (and be notified when future tutorials are published here on PyImageSearch), simply enter your email address in the form below!
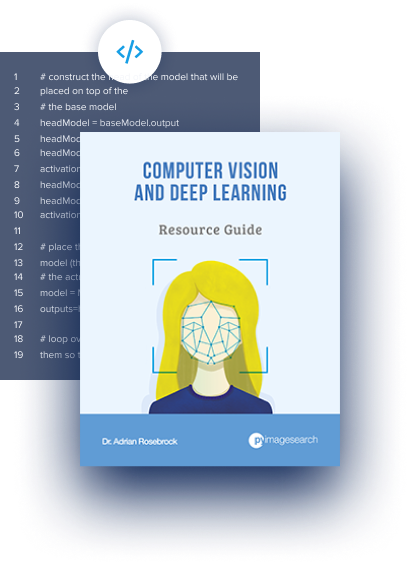
Download the Source Code and FREE 17-page Resource Guide
Enter your email address below to get a .zip of the code and a FREE 17-page Resource Guide on Computer Vision, OpenCV, and Deep Learning. Inside you'll find my hand-picked tutorials, books, courses, and libraries to help you master CV and DL!
The post Image Masking with OpenCV appeared first on PyImageSearch.